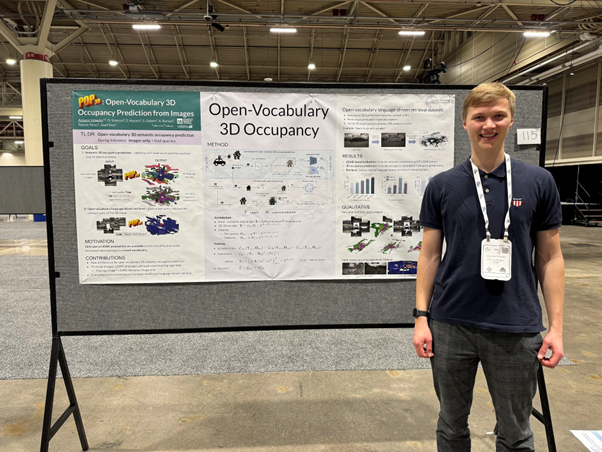
The work of Antonín Vobecký and Josef Šivic at CIIRC CTU, who, in collaboration with Valeo.ai, focus on developing machine learning models for better data analysis and especially analysis of images from self-driving cars, has been a great success. Their methods, which will improve the safety and reliability of autonomous driving, were presented at one of the world’s most important machine learning conferences – NeurIPS 2023 in New Orleans, USA. The seven-day conference brought together more than 16,000 attendees from among the world’s leading researchers and experts in artificial intelligence.
SOURCE: CIIRC CTU press release
In a recent paper, „POP-3D: Open-Vocabulary 3D Occupancy Prediction from Images“ (/doi.org/10.48550/arXiv.2401.09413) researchers presented a method that processes 360° camera images of a car’s surroundings as input and produces a 3D semantic map of the occupancy of the surrounding environment. In addition, the user can ask the system in natural language about the location of objects in this digital 3D space. The capability to make queries in natural language allows for semantic occupancy segmentation or text-driven search in 3D, both performed exclusively from 2D images taken by the vehicle’s cameras. Questions asked might be, for example, „Where is the plastic trash bin?“ or „Where is the black truck with the trailer?“. This work was published at the NeurIPS 2023 conference, one of the world’s most important machine learning conferences.
The overall motivation is to develop methods to search for corner case situations, which are safety-critical but often very rare in the driving recordings. The goal of the research is to enable the retrieval of these captured situations in huge files of petabytes of data by simply entering instructions in natural language. Once such situations are found, they can be used as additional training data to improve existing machine-learning models and algorithms for autonomous driving so that systems can better deal with such situations and, as a result, improve the safety and reliability of autonomous vehicles.